AI & Cybersecurity in Embedded Systems
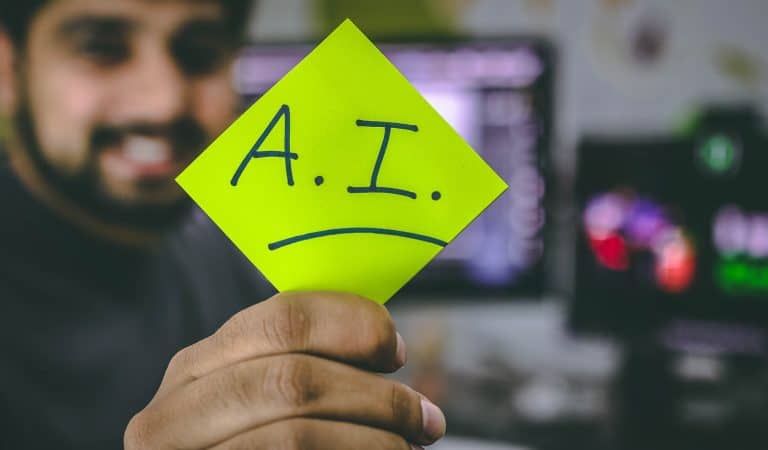
AI & Cybersecurity in Embedded Systems
Since its inception, Artificial Intelligence has been present in the minds of all players in the technology industry. AI is at the heart of people’s expectations for the future, from the potential it represents to the new possibilities it offers.
AI enables new products, services and business models to enter the market and opens up a new economy of data analysis. Data analysis, through AI, can improve business intelligence and helps businesses, individuals but also intelligent devices to make better decisions.
AI is having multiple impacts in many different industries, such as automotive and mobility, connectivity, smart cities or even critical infrastructure. For example, in the autonomous driving industry, AI can improve the effective safety of the vehicle. Having AI as an additional safety feature for a vehicle should reduce the risk of potential accidents, either through full control of the vehicle or through advanced driver assistance with sensors.
Critical hardware-based AI applications include Electronic Control Units (ECU) including Advanced Driver Assistants (ADAS) that exist in intelligent and autonomous vehicles, robotic control units or on-chip speech-recognition systems. The development of these AI applications rests solely on the shoulders of the OEM, without standard protection profiles or even a set of validation principals to verify the robustness of the AI implementation against cyber threats. Functional Security Requirements are the milestones for developing robust AI-centric embedded systems. The security challenge is not so much the detection rate capability of the AI hardware as it is, but the safety of the AI itself. In order to protect the integrity of the system, integrated cybersecurity has to protect against all disruptive technologies such as physical attacks, among which Fault Injection Attacks (FIA) that could cause security system integrity issues.
Security of Embedded Systems
Attacks against embedded AI systems are increasing due to the number of vulnerabilities discovered in these systems and the spread of AI implementations everywhere. Security is now more important than ever.
These attacks can be classified into three main categories:
- Adversarial inputs: Adding noise or modifying the input so that it is misclassified or completely ignored by the machine learning.
- Data poisoning: Targeting the training data where some of the samples are mislabeled so that the model learns false targets and compromises its own system.
- Model Stealing: Targeting the model itself by using invasive reverse engineering.
Threats and Vulnerabilities of AI
Embedded AI has already begun to be implemented in a wide range of use cases from data acquisition to autonomous driving.
The list of vulnerabilities is long when it comes to embedded AI production, as the data, the algorithm, the model architecture and also the system on which it is deployed can be a potential target for attacks. To solve this issue, cloud-based AI services have been introduced but they do not make the security foolproof as in certain real-time applications, the cloud network connectivity may not be reliable, making the system vulnerable. The AI core is therefore a requirement, which leaves the system with a target for potential attacks.
There are a number of targets on a chip or a board. For example, an attacker can modify any of the key parameters of the AI itself which will eventually lead the system to malfunction; these parameters (such as weight, bias, etc.) are called Critical Security Parameters of the embedded or edge AI system. Apart from physical attacks, an attacker can also create a pair of inputs and the corresponding response of the AI system to derive the functional metrics of the system so as to duplicate it and create adversarial inputs that could break the system.
Finally, some edge AI applications continue to learn from real-time data after deployment to improve their predictability. They are particularly vulnerable as they tend to update their detection parameters based on live inputs which makes it easier for the attacker to gain access to the system.
Using an integrated Secure Element (iSE) for OTT AI Protection
An iSE acts as the hard cover for sensitive information that resides directly in the SoC. It is used to protect the integrity of the data and to ensure that the information is only accessible to authorized users or applications. In OTT (Over The Top) AI systems, the most important features to be protected are the features and patterns learned by the AI during training phase. These features must be learned in a secure environment, without leaking sensitive information. An iSE will ensure that the AI parameters are safely stored so that computation and detection are performed in a secure environment and isolated from regular processes.
How can Secure-IC protect your AI system?
Secure-IC has developed a product called SecuryzrTM iSE to protect a system against the relevant threats identified in the threat model for AI systems. It offers a multitude of services throughout the lifecycle of the device such as key management, secure boot, cryptographic services to client processes, security monitoring and data protection.
SecuryzrTM iSE provides protection for data confidentiality and authenticity as well as a Secure Boot. Additional protections such as a Digital Sensor and an Active Shield for anti-tampering protection can be included.
With AI-based attacks becoming increasingly important, Secure-IC has also implemented a cutting-edge AI-enhanced analysis in its security evaluation tools. For advanced attacks, AI-based protection will be the best solution, therefore using Secure-IC’s Smart Monitor as well as real-time, hardware-based security policy should allow for enhanced security policy.
The main benefits of SecuryzrTM to protect AI from threats are:
- Embedded firmware is verified by the CatalyzrTM tool to be robust against information.
- Mixed design is checked against security issues with the VirtualyzrTM
- SecuryzrTM is ready for FIPS 140-3, CC EAL4+ and OSSCA certifications.
- SecuryzrTM is flexible. It can reach high performance or low surface area for AIoT and has many options in terms of services and protection.
- It is fully digital. Analog designs are not required as it based on standard cells.
Do you have questions on this topic and on our protection solutions? We are here to help.